Federated learning
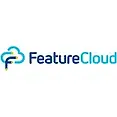
FeatureCloud
FeatureCloud is an all-in-one platform to RUN, DEVELOP & PUBLISH federated & privacy-preserving machine learning algorithms.
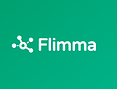
Tool for differential gene expression analysis
Flimma is the federated implementation of the popular differential expression analysis workflow limma voom. Flimma provides several advantages over the existing approaches for gene expression analysis. Unlike limma voom, Flimma by design preserves the privacy of the data in the cohorts since the expression profiles never leave the local execution sites. In contrast to meta-analysis approaches, Flimma is particularly robust against heterogeneous distributions of data across the different cohorts, which makes it a powerful alternative for multi-center studies where patient privacy matters.
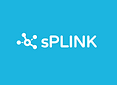
safe PLINK
sPLINK (safe PLINK) allows the federated, privacy-preserving analysis of GWAS data. It works on distributed datasets without exchanging raw data and is robust against imbalanced phenotype distributions across cohorts. Federated and user-friendly analysis with sPLINK, thus, has the potential to replace meta-analysis as the gold standard for collaborative GWAS.

Privacy-Aware-Time-to-Event Analysis
Partea (Privacy-Aware-Time-to-Event Analysis) is a federated-learning platform to perform time-to-event studies (survival analysis) on data that is distributed across different sites. The platform comes with a friendly user interface, does not require programming skills and makes it easy to collaborate with other institutions in the analysis of time-to-event data using Cox Proportional Hazards Model, Survival Curves or Log-rank tests. Through Federated Learning in combination with Privacy-enhancing technologies, such as differential privacy or secure multi-party computation, Partea makes sure that your sensitive data does never leave your local site while only insensitive model parameters are shared with the Partea server.